Microneedles (MNs) are an emerging technology for user-friendly and minimally invasive injection, offering less pain and lower tissue damage in comparison to conventional needles. With their ability to extract body fluids, MNs are among the convenient candidates for developing biosensing setups, where target molecules/biomarkers are detected by the biosensor using the sample collected with the MNs. MNs have been fabricated based on empirical data such as mechanical testing, and their physical parameters have been optimized through the trial-and-error method. While these methods showed adequate results, the performance of MNs can be enhanced by analyzing a large data set of parameters and their respective performance using artificial intelligence. Our lab has been applying finite element methods (FEMs) and machine learning (ML) models to determine the optimal physical parameters for MN designs in order to maximize the amount of collected fluid. The fluid behavior in a MN patch is simulated with several different physical and geometrical parameters using FEM, and the resulting data set is used as the input for ML algorithms including multiple linear regression, random forest regression, support vector regression, and neural networks. Decision tree regression (DTR) yielded the best prediction of optimal parameters. ML modeling methods can be utilized to optimize the geometrical design parameters of MNs in wearable devices for application in point-of-care diagnostics and targeted drug delivery.
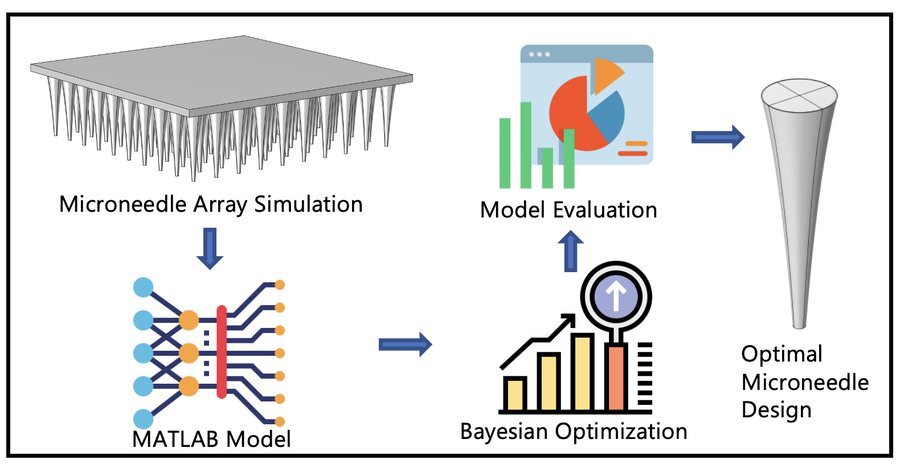
Published Papers: